Biased AI
- Christie Lam
- Nov 22, 2020
- 3 min read
Technology has come a long way since the first few generations. Now, people rely on technology for their everyday personal and work lives. At every turn, you will encounter a piece of technology with which you rely on or will interact with constanly. The healthcare industry is no different. The advancements in the technological world have led to technology seeping into different markets. Within the healthcare market, many doctors and hospitals, through artificial intelligence and machine learning, have begun using computer systems to perform tasks specialized doctors would. For example, some systems can diagnose skin cancer (in the department of dermatology), detect a stroke on a CT scan (in the department of radiology), and even notice cancers on a colonoscopy (in the department of gastroenterologist). Even though these technological advancements have leveled up our physicians and the care they can provide, many fear that these computer systems may make medicine more biased than it already is.

AI systems work on the basis of data, and with the data provided, it makes decisions. In essence, this is a good thing, but when given minimal data, it is unable to make accurate decisions. Statistics show that many of the AI algorithms that have been programmed with the data they are given do not accurately represent the whole population; more often than not, they perform less when presented with an underrepresented people group. Some examples of this include underperformance of chest exams due to a gender imbalance of the data and worse skin cancer detection on darker skin. Healthcare is an industry with high stakes, if someone or something makes the wrong call or decision, a life may be lost. Because of this, it is important that these sets of data hold information from various demographics which currently is not the case.
Despite the severity of this issue, the solution is not simple as there are many factors that come into play. One of the main roadblocks is the laws put in place to protect data and the sharing of this data. Medical data includes a lot of personal information that many may not want others to have access to. Because of this, there are many hospitals that retract from sharing their data in fear of losing their patients to other hospitals. Another issue that one may encounter when trying to solve the biases in AI is some unexplainable technological barriers. There have been instances where the computer systems are unable to interpret the data given. In one case, an AI system at a hospital recommended hospitalization for a patient with pneumonia but would clear that same person if they had both asthma and pneumonia.
With the COVID-19 pandemic and the vaccine coming out, this issue is more prominent than ever. Thousands of lives are lost each day and it is important that we do not misdiagnose any patient. One of the lead companies developing the coronavirus vaccine delayed their release because they sought to recruit more participants from different demographics in their testing. This is a clear example of how progress is being made to address and correct these issues. In another instance, the 2019 NeurIPS conference gave a workshop that promoted fairness in machine learning in the healthcare industry. Within the workshop, there were papers that assessed algorithmic fairness, discovering proxies, and calibrate algorithms for subpopulations. Additionally, some organizations and corporations have started incorporating inclusivity into the data-gathering process, training, and the testing of algorithms.
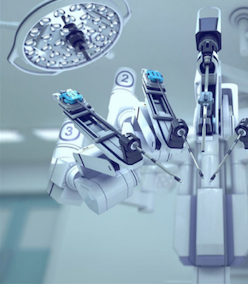
Biases in general are a tough concept to attack, and when technology is involved, it becomes even more difficult. There are so many factors that come into play such as a lack of diversity in developers and implicit biases. No amount of training can guarantee the elimination of bias. Additionally, with the difficulty in achieving data, it is difficult to find solutions. In order to be able to tackle biases and guarantee that future algorithms are powerful and fair, the correct infrastructure must be built in order to provide and deliver diverse data to train these computer systems. One can and should no longer settle with data that leads to disaster filled consequences.
Comments